It has really just been over a year since the release of ChatGPT, a milestone not so much in the abilities of Generative AI but more of a public announcement of the astounding capabilities of this technology.
A year on, the promise is stronger than ever, and the horizon of possibilities seems infinite, compelling leaders in various domains to embed Generative AI into their processes and harness its potential. Some predictions indicate that Generative AI could elevate the global GDP by 7% and substantially augment productivity growth in the forthcoming decade. However, how much credence can we place in these optimistic projections?
This consideration is crucial, given the prevalent reservations and uncertainty among the leadership. The divide exists not just inter-corporate but intra-corporate as well. A research report by the consulting group BCG, surveying 2,000 global leaders based on BCG's Digital Acceleration Index, showcased that a significant majority, over 50%, remain cautious about adopting Generative AI. (See Exhibit 1 for details.)
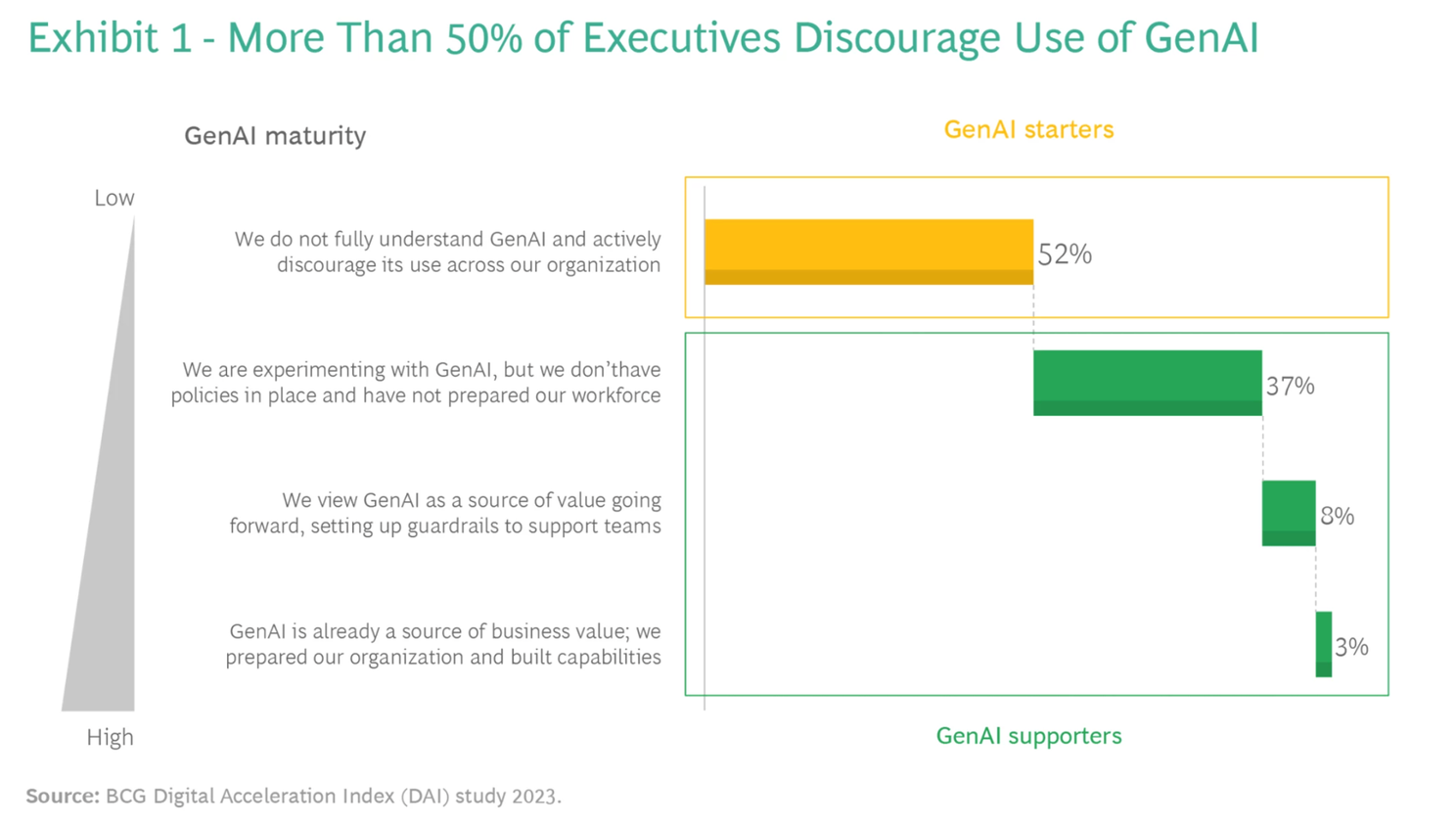
When talking to business leaders about Generative AI, the concerns they express usually fall into one of three buckets:
- Reliability - Is there a risk of the Language Model (LLM) generating false or misleading information?
- Privacy - Will our sensitive corporate data be exposed if we use a public LLM?
- Governance - Can we ensure explainability, traceability, and audit-ability when using these models?
While these are valid questions, the nascent nature of the field means there's a scarcity of enterprise-level guidance on how to address them. Let's delve into these issues.
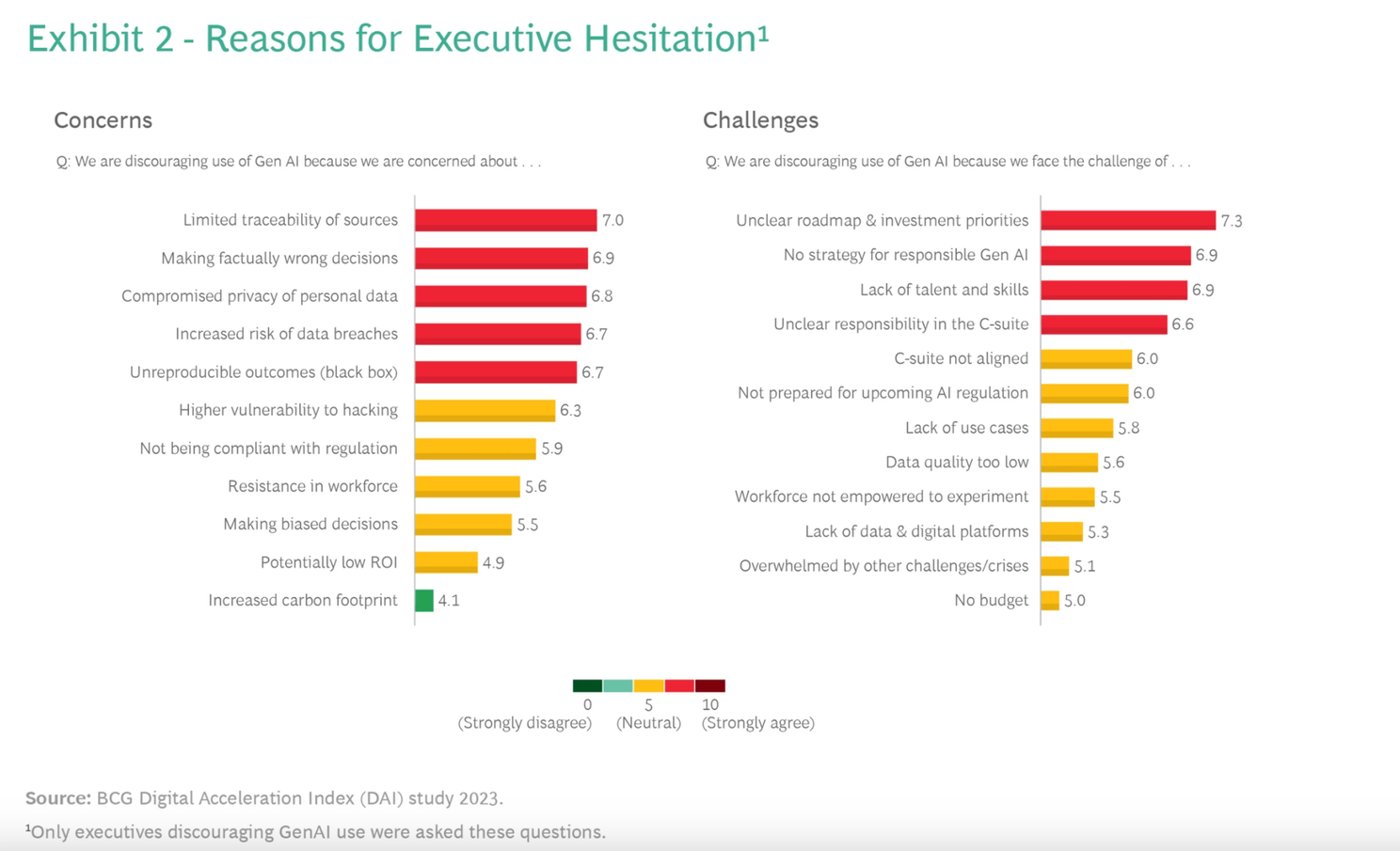
The Imperative for AI Operations
To understand the necessity of AI Operations (AI Ops), consider a more conventional technology scenario: If your organisation were contemplating a switch from one database system to another, questions like "Will the database misplace my data?" would likely be considered absurd. Why? Because there are established operational procedures—like testing, Continuous Integration/Continuous Deployment (CI/CD), and monitoring—that ensure the reliability and integrity of database systems.
Similarly, the application of Generative AI in an enterprise context requires a robust operational framework. Just as you wouldn't deploy a new database without rigorous testing and governance, you shouldn't deploy a Language Model without a corresponding set of operational procedures. This can come in the form of an AI Ops platform that uses several tools and processes in order to realise the tremendous value that Generative AI can offer.
Let’s look at each of the above areas of concern and how we can address these with a robust AI Ops roadmap.
Reliability:
What do we mean by this? Well, we have all read the stories about a lawyer in the US who built his whole case on fabricated case law made up by a large language model. The colourful term “hallucination” gets bandied around when relating to LLMs exhibiting this behaviour. But was this lawyer using a large language model as part of his firm’s AI operations stack where the output could be examined and audited or where his prompts could be audited? No, he was just doing research in ChatGPT and using the LLM’s corpus of knowledge or parametric knowledge for research.
So how might this lawyer have received a better and less costly result with his misadventures in AI? Well, if his firm had provided a research tool that was LLM-based but limited to internal verified case law, a verified knowledge-base, this “hallucination” would not have happened.
So what tool in the AI Ops toolkit could have achieved this? Well, there are a few but the most cost-effective and implementable of these is an architectural pattern called Retrieval Augmented Generation (RAG).
RAG acts as a bridge, connecting LLMs to real-time or domain-specific data from external databases or even your own company's proprietary databases. This connection is crucial as it arms the LLM with the most current and relevant data when formulating responses, significantly reducing hallucinations.
Here are a few ways RAG is redefining the Generative AI landscape for businesses:
- Up-to-date Information: Stay ahead with the latest market trends and business data as RAG ensures your AI applications are drawing from the most recent information.
- Domain-Specific Knowledge: Tailor your AI's responses to your industry's unique nuances, enhancing its utility and relevance.
- Audit-ability: Trace back the AI-generated insights to their original sources, promoting transparency and trust.
- Leveraging Proprietary Data: Enrich your AI's knowledge base with your company's in-house data, unlocking new levels of insights and decision-making prowess.
This last one is the key. By creating a pipeline of specific information, the LLM is not using its own (parametric) knowledge at all, instead, it is using the rich data you have in your organisation. There is still room for error, but the LLM can be completely prevented from fabricating information as we witnessed with our unfortunate lawyer and his chatGPT misadventures.
RAG is not just a technical upgrade; it's a step towards making AI a reliable partner in driving business growth and innovation. As business leaders, embracing RAG could be a strategic move to refine your AI operations and deliver better value to your stakeholders. As mentioned this is not the only tool for reliability, but it's certainly one of the most powerful.
Data Privacy
You might ask - Will our sensitive corporate data be exposed if we use a public LLM? Perhaps you have heard that LLMs are training on the data you send.
This all depends on how you have structured your AI Operations. This is like asking if I put my data in a database, is it safe? Well, if you put company data in a private AWS or Azure instance then with proper precautions most companies would be satisfied that this is safe.
Large language Model companies have been trying to address these questions along with large players such as Microsoft’s OpenAI Azure platform as well as the newly released AWS Bedrock with access to corporate-level LLMs such as Cohere and Claude 2.
These infrastructures allow access to top LLMs but without company data being used to train models. OpenAI has also released an Enterprise product which gives the same guarantees.
Managing internal privacy policies in this area is crucial; this includes providing safe tools for employees to make productivity gains from LLMs. This lowers the risk that employees are uploading sensitive data to ChatGPT or other consumer AI products.
An abolition of Generative AI is not an adequate privacy policy, it will instead stymie growth and push employees to bend the rules when they can, causing further risk.
The best ways to ensure data privacy in an organization with use of Generative AI are:
- Have a clear informed privacy policy which takes into account the level of sensitivity of the information
- Evaluate the technology for specific tasks or classes of tasks
- Have checks and balances built into your AI Ops platforms
- Where possible use platforms such as AWS Bedrock, Azure OpenAI service or OpenAI Enterprise or models that are for enterprises such as Cohere.
- Keep humans in the loop (HITL)
Governance
Another well-worn trope about Generative AI is that there is no “Explainability” with its output and therefore no accountability about what it might output at any one time.
In other words, we cannot look into the model like we would an algorithm and see why the model said “X” instead of “Y”, this on the face of it is true. We cannot analyse a prompt and output like we would a SQL query and a query output. This does not mean that there cannot be governance or accountability for what Generative AI or an LLM might output. We merely have to approach the problem in a different way. Here are some methods that should be in our AI Ops toolkit.
- Data Source Constraints - This is the same method to stop hallucination: Controlling what data on which the LLM is using to reason and answer questions, offers a different kind of explainability. This can be achieved in very fine detail.
- Data Source citation - Each output should cite the data source (usually from the vector store). This means output can be understood and prompts tweaked.
- Prompt Library - A library of prompts with versioning, usage and linking them to audit logs of outputs means all LLM usage in an organisation is traceable.
- Output Audit - All LLM output in critical areas of an organization should be logged and linked with the prompt that was used and the prompt.
- Human In The Loop (HITL) - In critical systems where there is a lot of backend AI automation, QA systems should be in place to keep human checks on audits and outputs.
Hype Cycle Fatigue
As generative AI technology increasingly permeates public discourse there may be a temptation on the part of some business leaders to dismiss this as another hype cycle.
They may remember three years previously having to sit through presentations on how distributed ledgers and Web 3.0 would change everything, and think sitting out this latest hype could save some precious hours. Not to dunk on Web 3.0 but its applications were niche and often a solution in search of a problem where enterprise technology was concerned.
Generative AI, by contrast to Web 3.0 is a Swiss army knife that can be immediately applied to many different use cases across an organisation for instant productivity gains.
As we’ve examined above, the issue is not functionality or implementation of the technology but instead creating the platform to make these new and useful tools conform to our expectations of reliable output, privacy concerns and corporate governance.
Key Questions
While the "wait and see" approach may have been justifiable in 2023 as the industry fine-tuned its capabilities, the landscape is shifting rapidly. The year 2024 is set to witness a surge in the enterprise adoption of Language Learning Models (LLMs) across various departments. The emerging role of the AI Operations Director will be crucial in orchestrating LLMs to ensure reliability, privacy, and governance.
The key question for leaders is: How important is Generative AI going to be in my industry? Could new competition catch up and overtake us in our industry by using these technologies? Could we reach new markets, bring more value and create new products with this technology?
AI Operations has to start somewhere
AI Operations is not merely an operational appendage; it's a strategic imperative. Firms that invest in solid AI Ops infrastructure today aren't just sidestepping potential pitfalls—they're setting the stage for rapid growth and innovation. The quicker businesses realize this, the faster they can harness the full, transformative potential of AI in a responsible and impactful way.
Now is the time to assess your organization's AI Ops readiness and take proactive steps to integrate intelligent automation across your enterprise. The advancements in 2024 won't wait—prepare now to navigate the complexities ahead. If you would like to talk to us about your strategy, AI Operations and how you can start with a quick proof of concepts get in touch with us to have a chat.